ML Techniques and DevOps For Increased Service Reliability
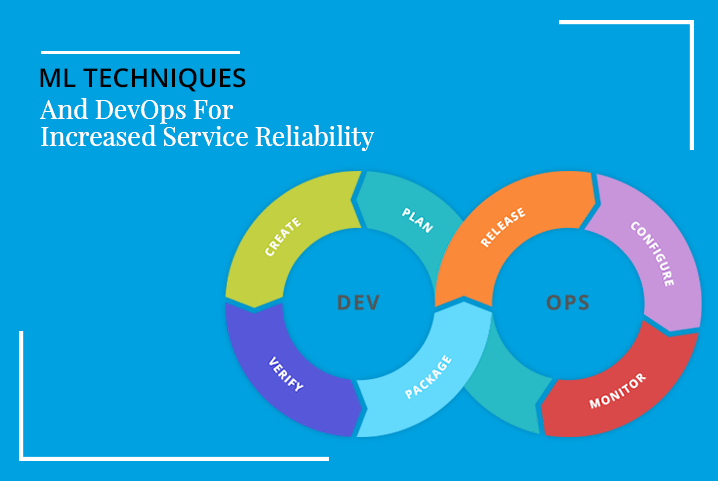
The benefits of DevOps for enterprises are beyond measure. Particularly, in today’s increasingly competitive environment where businesses cannot just afford to be good anymore, DevOps provides the perfect competitive edge that lets businesses reduce complexity and introduce agility in service or product delivery.
However, the benefits are not limited to products alone, DevOps helps streamline enterprise operations for improved collaborations among cross functions for much efficient collaboration and more improved flexible environment driving product/service innovation.
With such huge benefits, a legitimate question is then what more can Machine Learning add to the all attracting DevOps enterprise benefit deck?… Well, a one-word answer would be ‘Consistency’. It’s like DevOps helping you to hit Home run once but Machine Learning tells you to how to hit it again and again.
Handling Data Volumes:
While DevOps is a practice for ensuring Continuous software delivery with equal efficiency for greater cross-functional innovation, ML equips organizations to handle large data volumes with accuracy and speed beyond the scope of traditional engineers. What this means is that ML overtime upon a complete overview of the process can employ AI and Deep learning tools for handling a large volume of diverse datasets at a speed unmatched by a team.
With improved and efficient data classification ML helps DevOps environment to strengthen key customer retention strategies like personalization, security, product recommendations, fraud detection etc.
Improving Production Accuracy
Like any It solutions, DevOps solutions also do not guarantee 100% success. They are susceptible to failures too. ML can help minimize this failure probability by a considerable factor.
It can be used to map known datasets for a better and diverse range of forecasts. ML can be the perfect guiding light of enterprises to foresee any impending product/service failure. Further, it can be also used to introduce structural and organizational changes for improving performance. Lastly, it can help Ops teams with a much greater response time during outages.
Improved Application Reliability
Security remains a primary concern for enterprises today. Especially identifying the bad apples from the good ones can be a daunting task. Especially in a network environment buzzing with user activity, the probability of identifying a suspicious behavior pattern becomes highly unlikely. But ML takes a big load of this work from the Ops team. By reporting anomalies on a real-time basis, it helps better secure sensitive data as well as better fence routines, deployments, execution routines.
Managing Production
Continuous service delivery is the prime objective for any DevOps environment. The shorter turnaround times mean that the time request documentation and coding is significantly less when compared to a normal environment. ML can help differentiate the normal pattern from the abnormal patterns with a certain amount of accuracy unassociated with a normal DevOps environment. Especially in a production environment, the large data volumes make it beyond the scope of cross-function to identify memory leaks or race conditions.
The Perfect Tranquiliser
Many compare a DevOps investigation environment to being on steroids. Such is the agile development demand that developers may be required to change and deploy codes more than a couple of times within a single day. As such there are good changes that some problems might escape the prying eyes unnoticed. This adversely affects the end user or consumer experience and by the time any solution is put into place, the damage has already been done as far as user experience and satisfaction are concerned.
Developers have to scrutinize large volumes of infrastructure and application log files. The application log files are particularly taxing. Inconsistencies can be as hard to find a hen’s teeth.
But Machine Learning can prove to be effective beyond measure in this regard. ML can help bring efficiencies and accuracy in log management and analysis for identifying inconsistencies with greater accuracy than ever.
The above are just a few of the manifold advantages that integrating ML techniques can introduce greater efficiency to a DevOps based service delivery environment. DevOps helps an enterprise to automate agile product development and deployment. Pratham has helped enterprises of all scales and size to leverage DevOps for increased automation in workplaces based on their market needs.
- Older
- Newer