SalesForce Einstein Discovery
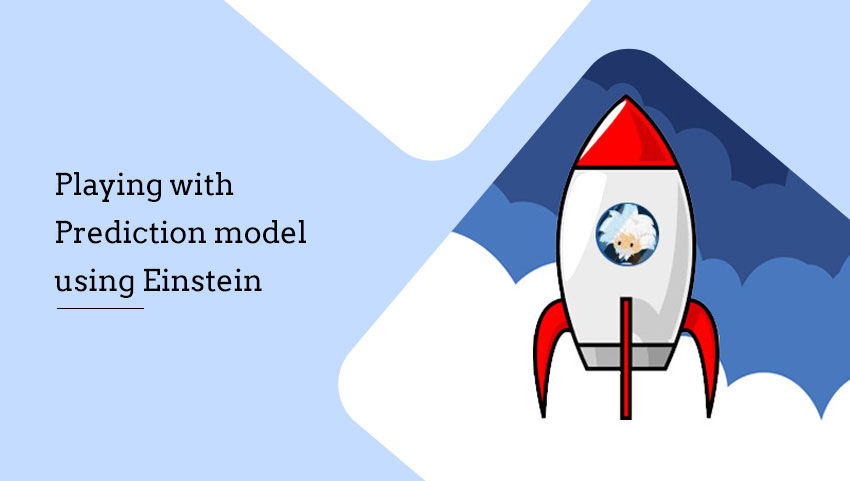
In Supervised Machine Learning, we do either ‘Estimation of a Value’ OR ‘Classification into a category’. Naturally, the predicted variable values are then Numerical or Categorical respectively. Here in this BLOG we explore how in peculiar cases, can both of them might be tried basis final expectation from the Salesforce Einstein prediction model.
SalesForce Einstein Discovery Story also uses both of these only, via its homegrown auto ML & comprehensive AI functionalities. For Classification, we can only have BINARY classification (max. 2 classes) currently. For Estimation, we are able to set predicted value as only whole numbers optionally.
Data & Business Case:
Single Dataset having Orders & Order Reviews (made by Customers) details, with a Review Score provided from value 1 to 5 (1 being Worst, 5 being Best).
We consider the REVIEW SCORE as the KPI here, and naturally would look to maximize it for future orders.
Run I : ESTIMATING a Value (Linear Regression)
When the dataset was uploaded, Review score is considered as Numeric or a ‘Measure’ in Einstein Analytics. Hence we simply look to maximize this value here for generating model. Also since the values here can NOT be in fractions; we select the concerned checkbox also.
It may be inferred here that this is a sorts of multi-class classification here, I leave that as open thought for readers here to further delve! 🙂
Output: A very poor accuracy model is generated, with R-squared below 30% on Validation set. Although the narrative insights generated can still be highly useful!
Run II: Classification into a category
For this, we can first transform this dataset via the bucketing option available in RECIPE (1–3 as BAD, 4–5 as GOOD)
We can now run the model again on this transformed dataset, with KPI selection as:
Output: The accuracy here comes out to be fair and acceptable (above 75%).
Selecting the SalesForce Einstein Type of Model:
By first thought, we might think to select classification after transformation as giving much better accuracy.
However, the business requirement might be such that we need to predict review scores of future ORDERS, when say they are still in transit. Hence, the ESTIMATION would still be applicable in that case, provided accuracy was better if not more than classification. Also since we are using Einstein Discovery Story and hence not just getting future predictions (like by Einstein Prediction Builder); we can actually use the hindsight’s provided to identify the root causes (predictor variables) driving a particular score value, individually.
For CLASSIFICATION, other than getting better accuracy (bit obvious also as 5 values reduced to 2 categories!), we may have a business requirement where they need to take action on lower score (BAD) Orders, and need that to be available at its record level; by usage of EINSTEIN NEXT BEST ACTION like:
(*This is an indicative image only)
- Older
- Newer